Introduction#
Machine Learning-Based Techniques for observation and administration are especially suitable for sophisticated network infrastructure operations. Assume a machine learning (ML) program designed to predict mobile service disruptions. Whenever a network administrator obtains an alert about a possible imminent interruption, they can take bold measures to address bad behaviour before something affects users. The machine learning group, which constructs the underlying data processors that receive raw flows of network performance measurements and store them into such a Machine Learning (ML)-optimized databases, assisted in the development of the platform. The preliminary data analysis, feature engineering, Machine Learning (ML) modeling, and hyperparameter tuning are all done by the research team. They collaborate to build a Machine Learning (ML) service that is ready for deployment (Chen et al., 2020). Customers are satisfied because forecasts are made with the anticipated reliability, network operators can promptly repair network faults, and forecasts are produced with the anticipated precision.
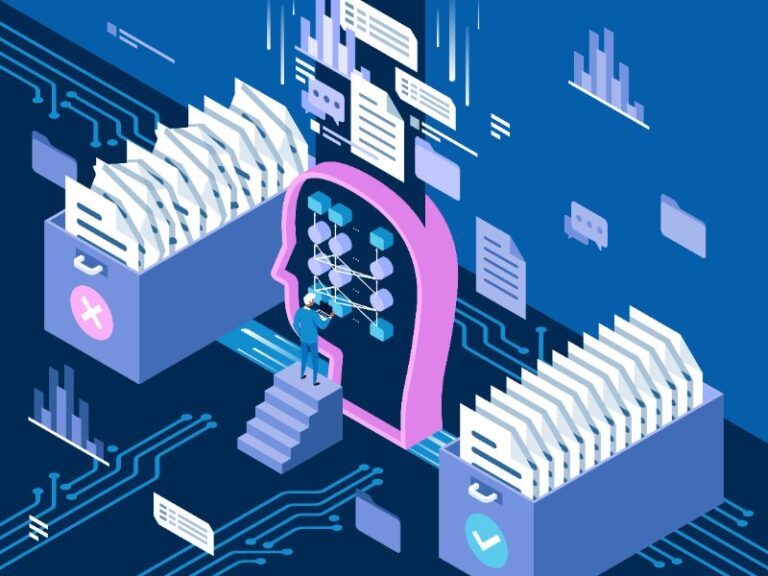
What is Machine Learning (ML) Lifecycle?#
The data analyst and database administrators obtain multiple procedures (Pipeline growth, Training stage, and Inference stage) to establish, prepare, and start serving the designs using the massive amounts of data that are engaged in different apps so that the organisation can take full favor of artificial intelligence and Machine Learning (ML) methodologies to generate functional value creation (Ashmore, Calinescu and Paterson, 2021).
Monitoring allows us to understand performance concerns#
Machine Learning (ML) models are based on numbers, and they tacitly presume that the learning and interpretation data have the same probability model. Basic variables of a Machine Learning (ML) model are tuned during learning to maximise predicted efficiency on the training sample. As a result, Machine Learning (ML) models' efficiency may be sub-optimal when compared to databases with diverse properties. It is common for data ranges to alter over time considering the dynamic environment in which Machine Learning (ML) models work. This transition in cellular networks might take weeks to mature as new facility units are constructed and updated (Polyzotis et al., 2018). The datasets that ML models consume from multiple data sources and data warehouses, which are frequently developed and managed by other groups, must be regularly watched for unanticipated issues that might affect ML model results. Additionally, meaningful records of input and model versions are required to guarantee that faults may be rapidly detected and remedied.
Data monitoring can help prevent machine learning errors#
Machine Learning (ML) models have stringent data format requirements because they rely on input data. Whenever new postal codes are discovered, a model trained on data sets, such as a collection of postcodes, may not give valid forecasts. Likewise, if the source data is provided in Fahrenheit, a model trained on temperature readings in Celsius may generate inaccurate forecasts (Yang et al., 2021). These small data changes typically go unnoticed, resulting in performance loss. As a result, extra ML-specific model verification is recommended.
Variations between probability models are measured#
The steady divergence between the learning and interpretation data sets, known as idea drift, is a typical cause of efficiency degradation. This might manifest itself as a change in the mean and standard deviation of quantitative characteristics. As an area grows more crowded, the frequency of login attempts to a base transceiver station may rise. The Kolmogorov-Smirnov (KS) test is used to determine if two probability ranges are equivalent (Chen et al., 2020).
Preventing Machine Learning-Based Techniques for system engineering problems#
The danger of ML efficiency deterioration might be reduced by developing a machine learning system that specifically integrates data management and model quantitative measurement tools. Tasks including data management and [ML-specific verification] are performed at the data pipeline stage. To help with these duties, the programming group has created several public data information version control solutions. Activities for monitoring and enrolling multiple variations of ML models, as well as the facilities for having to serve them to end-users, are found at the ML model phase (Souza et al., 2019). Such activities are all part of a bigger computer science facility that includes automation supervisors, docker containers tools, VMs, as well as other cloud management software.
Data and machine learning models versioning and tracking for Machine Learning-Based Techniques#
The corporate data pipelines can be diverse and tedious, with separate elements controlled by multiple teams, each with their objectives and commitments, accurate data versioning and traceability are critical for quick debugging and root cause investigation (Jennings, Wu and Terpenny, 2016). If sudden events to data schemas, unusual variations to function production, or failures in intermediate feature transition phases are causing ML quality issues, past and present records can help pin down when the problem first showed up, what data is impacted, or which implication outcomes it may have affected.
Using current infrastructure to integrate machine learning systems#
Ultimately, the machine learning system must be adequately incorporated into the current technological framework and corporate environment. To achieve high reliability and resilience, ML-oriented datasets and content providers may need to be set up for ML-optimized inquiries, and load-managing tools may be required. Microservice frameworks, based on containers and virtual machines, are increasingly widely used to run machine learning models (Ashmore, Calinescu, and Paterson, 2021).
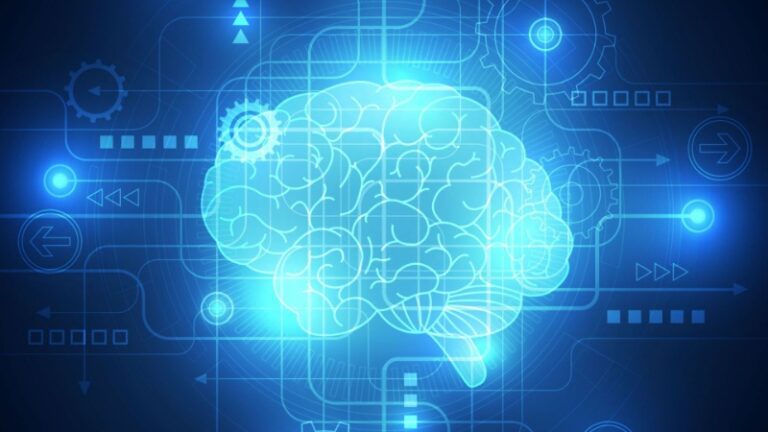
Conclusion for Machine Learning-Based Techniques#
The use of Machine Learning-Based Techniques could be quite common in future communication designs. At this scale, vast amounts of data streams might be recorded and stored, and traditional techniques for assessing better data and dispersion drift could become operationally inefficient. The fundamental techniques and procedures may need to be changed. Moreover, future designs are anticipated to see an expansion in the transfer of computing away from a central approach and onto the edge, closer to the final users (Hwang, Kesselheim and Vokinger, 2019). Decreased lags and Netflow are achieved at the expense of a more complicated framework that introduces new technical problems and issues. In such cases, based on regional federal regulations, data gathering and sharing may be restricted, demanding more cautious ways to programs that prepare ML models in a safe, distributed way.
References#
- Ashmore, R., Calinescu, R. and Paterson, C. (2021). Assuring the Machine Learning Lifecycle. ACM Computing Surveys, 54(5), pp.1–39.
- Chen, A., Chow, A., Davidson, A., DCunha, A., Ghodsi, A., Hong, S.A., Konwinski, A., Mewald, C., Murching, S., Nykodym, T., Ogilvie, P., Parkhe, M., Singh, A., Xie, F., Zaharia, M., Zang, R., Zheng, J. and Zumar, C. (2020). Developments in MLflow. Proceedings of the Fourth International Workshop on Data Management for End-to-End Machine Learning.
- Hwang, T.J., Kesselheim, A.S. and Vokinger, K.N. (2019). Lifecycle Regulation of Artificial Intelligence– and Machine Learning–Based Software Devices in Medicine. JAMA, 322(23), p.2285.
- Jennings, C., Wu, D. and Terpenny, J. (2016). Forecasting Obsolescence Risk and Product Life Cycle With Machine Learning. IEEE Transactions on Components, Packaging and Manufacturing Technology, 6(9), pp.1428–1439.
- Polyzotis, N., Roy, S., Whang, S.E. and Zinkevich, M. (2018). Data Lifecycle Challenges in Production Machine Learning. ACM SIGMOD Record, 47(2), pp.17–28.
- Souza, R., Azevedo, L., Lourenco, V., Soares, E., Thiago, R., Brandao, R., Civitarese, D., Brazil, E., Moreno, M., Valduriez, P., Mattoso, M., Cerqueira, R. and Netto, M.A.S. (2019).
- Provenance Data in the Machine Learning Lifecycle in Computational Science and Engineering. 2019 IEEE/ACM Workflows in Support of Large-Scale Science (WORKS).
- Yang, C., Wang, W., Zhang, Y., Zhang, Z., Shen, L., Li, Y. and See, J. (2021). MLife: a lite framework for machine learning lifecycle initialization. Machine Learning.