Computer Vision and Machine Learning For Healthcare Innovation
Computer vision is transforming healthcare by enabling advanced imaging analysis to aid in diagnosis, treatment, and patient care.
Half of the world's population does not have access to quality healthcare, and many people are driven into poverty. Over \$140 billion annually would be invested to achieve health-related sustainable development goals. There is a significant financing space for health IT, digital IT, and AI to help close the healthcare gap in developing countries.
As much as \$2 billion was invested in 2018 by health startups and IT businesses specifically to use AI technology. These funds account for a significant chunk of the total capital allocated to artificial intelligence projects.
This series focuses on how computer vision and deep learning are being used in industrial and business environments on a grand scale. This article will discuss the benefits, applications, and challenges of using deep learning methods in healthcare.
#
Benefits of Computer Vision and Machine Learning for Healthcare Innovation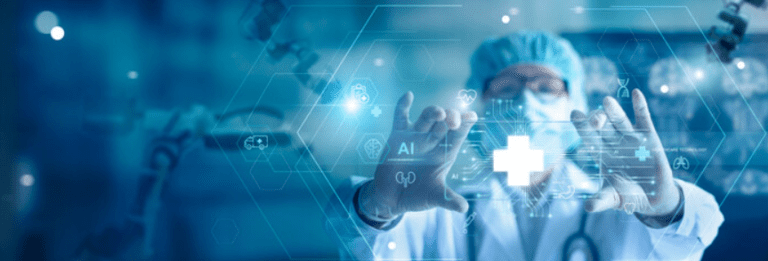
#
Unlocking Data for Health ResearchPlenty of new data is becoming readily available in the healthcare industry. This opens up vast opportunities for study and improvement. Mining and properly analyzing this data may improve clinical outcomes, earlier illness identification, and fewer preventable missteps.
However, getting enough high-quality, well-structured data is complex, especially in developing countries. Businesses use analytics and data cleansing methods to increase data interoperability. Also, this helps them to pave the way for valuable predictions that improve medical outcomes and decrease related issues.
Besides organizing data for analysis, using ML in large data settings can better connect patients. However, a business can accelerate the development of new drugs and pinpoint the most successful treatments in the life sciences.
#
Healthcare EfficiencySaaS businesses automate numerous activities. This includes arranging follow-up appointments and using patient data like consultation notes, diagnostic images, prescription prescriptions, and public information. This software-as-a-service (SaaS) offerings are revolutionizing developing countries by addressing problems like a need for qualified medical professionals and an absence of information about the quality of treatment.
#
Reaching Underserved CommunitiesEmerging countries use digital health technologies for health information, diagnosis, and treatment. Digital healthcare solutions can efficiently assist marginalized people, particularly in rural areas.
Machine learning may diagnose and suggest a specialist using public data and customer information. After reviewing the specialist's qualifications and user reviews, the patient may schedule a chat or call and pay online. In rural and low-income regions with few 3G-4G access and smart devices, SMS healthcare advice is a game-changer.
#
Applications of Computer Vision and Machine Learning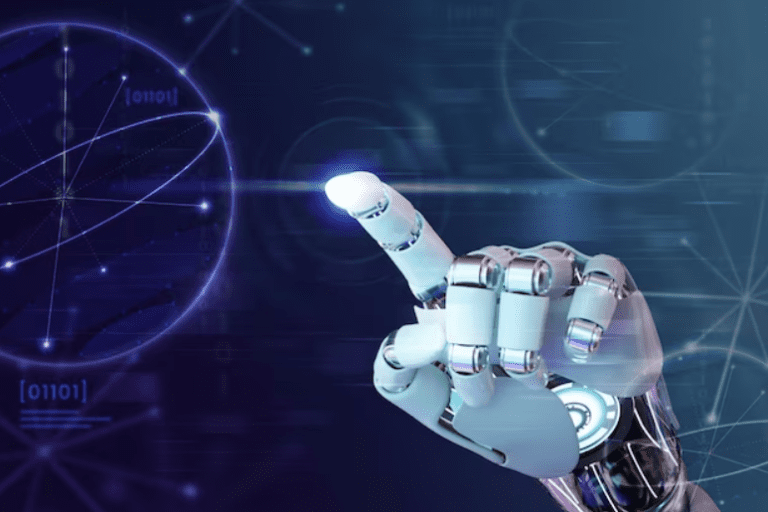
#
1. Medical Research in Genetics and GenomicsAI may help medical researchers discover drugs, match research studies, and find successful life-science remedies by analyzing important, complex information. AI can help researchers find disease-causing variations in genes and predict therapy outcomes.
By identifying patterns, AI can help us understand how human physiology reacts to drugs, viruses, and environmental variables. Machine learning algorithms may also analyze DNA sequences to predict the possibility of a disease based on data trends.
#
2. Medical Imaging and Radiology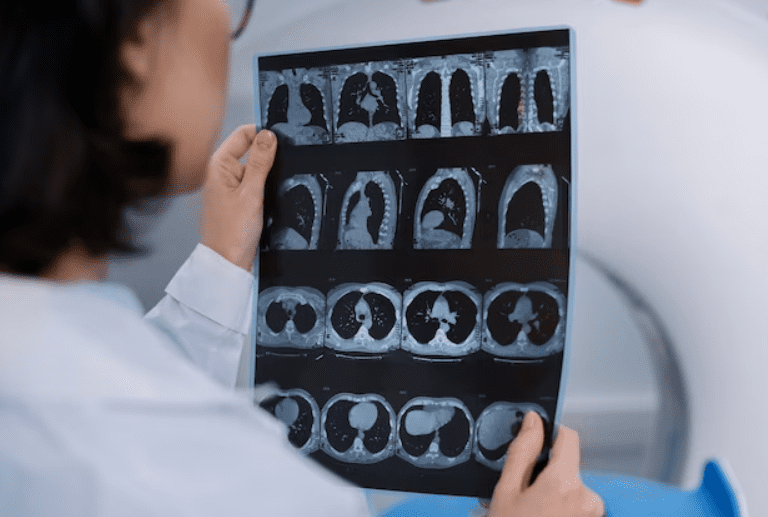
Machine learning and deep learning have improved radiology breast cancer diagnosis and CT colonography polyp identification. Deep learning algorithms can automatically extract and classify pictures rapidly, helping neuroimaging methods like CT and MRI diagnose strokes.
AI algorithms based on super-resolution methods may improve scan quality, which is generally inadequate owing to time restrictions in stroke patient management. AI can automatically identify tumors and enhance TB detection using X-ray and MRI data. AI can also use PET data to diagnose Alzheimer's early.
#
3. PathologyDigital pathology has created large volumes of data that may be utilized to teach AI frameworks to recognize trends and ease the global pathologist shortage. AI can automate hard and time-consuming activities like object quantification, tissue categorization by morphology, and target identification, helping pathologists.
AI may also compute personalized therapies, reduce the chance of misdiagnosis and drug errors, and encourage telepathology by permitting remote consultation with specialized pathologists. Finally, AI can identify visible signs like tumor molecular markers.
#
4. Mental HealthMental health management needs interaction between patients and providers. To enhance this connection, NLP and machine learning can collect and adapt to new facts. Virtual assistants, chatbots, and conversational agents can simulate human-like presence and help in searching online support communities, diagnosing major depressive disorder, and delivering cognitive behavioral therapy to individuals with depression and anxiety.
Moreover, virtual agents can serve as moderators of online communities for youth mental health when human moderators are unavailable. These agents can analyze participant posts' sentiments, emotions, and keywords to suggest appropriate steps and actions.
#
5. Eye CarePoint-of-care diagnostics using AI can replace visual software. Deep learning distinguishes healthy and AMD-afflicted eyes. It automatically predicts cardiovascular illness from retinal fundus images, evaluates age-related macular degeneration, checks for glaucoma, and diagnoses cataracts.
#
Some Challenges Faced While Using AI in HealthcareThe following are the key risks and challenges associated with using AI in the healthcare industry:
- Data privacy and security concerns.
- The effectiveness of AI may be limited for data that are difficult to obtain or rare.
- AI systems typically operate as black-box decision-makers, making it challenging or even impossible to understand the underlying logic that drives the outputs generated by AI.
- The system's insensitivity to impact means prioritizing making accurate decisions, even if it results in missed or overdiagnosis.
- Legal and regulatory challenges.
- Integration with existing healthcare systems.
- Limited accessibility to AI-based healthcare solutions for underserved communities.
- Technological limitations and the need for continuous monitoring and maintenance.
Hence, healthcare businesses must keep these issues in mind while integrating AI into their regular systems.
#
ConclusionSignificant investments are being pumped into the health technology and artificial intelligence industries to fill the gaps in healthcare services in growing countries. Artificial intelligence has shown some encouraging outcomes in several medical sectors, including radiology, medical imaging, neurology, diabetes, and mental health.
AI may assist in drug development, match patients to clinical trials, and uncover successful life-science solutions, all areas in which the medical research community can benefit. AI does this by analyzing and recognizing patterns in big and complicated datasets.
However, some challenges must be overcome to integrate AI successfully into the healthcare industry.