How Can Financial Enterprises Benefit From Private 5G Network Architecture?
Private 5G is a special framework of 5G designed for big organizations to benefit from high speed, low latency, and seamless connectivity. Read the full article to know about the business benefits of 5G.
#
IntroductionThe Fifth Generation Cellular network or 5G is not only going to change the lives of individual people but it is also going to revolutionize how financial enterprises control their data in the near future. It is better than 4G in every aspect whether it is speed or connectivity. Its fast speed, seamless connectivity, and low latency make it the perfect option for big enterprises.
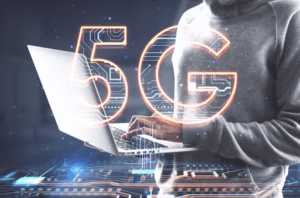
Private 5G will be very beneficial for large enterprises with thousands of employees and terabytes (TB) of data. To get a better understanding of private 5G architecture and its benefits, read the article till the end.
#
What is Private 5G?A private 5G/enterprise 5G is a private network setup by large organizations to work more efficiently. Large enterprises like Google, Facebook, Twitter, and many other organizations with thousands of employees and millions of terabytes of data use this network to benefit from low latency, fast speed, and seamless connectivity features.
Unlike 4G, 5G can handle millions of users in the same area. Moreover, it also can transfer big chunks of data in seconds. Different organizations use different CBRS spectrums to build their own network.
A private 5G network requires its users to set up small cellular towers built close together like wifi access points to transfer data efficiently. This type of network architecture will help universities, big plants, and warehouses to work more efficiently.
#
Benefits of Private 5GIt provides many amazing features that can benefit organizations in different ways.
Firstly, the data security feature it provides is amazing. Organizations will have full control over everything that happens because they don't need to connect to a telecom now.
Apart from security, this network architecture is also cost-effective. No cables are needed, you can now get a fast and reliable wireless connection that provides seamless connectivity.
It also provides various customizations to organizations to build an infrastructure like Micro Slicing. These customizations are not available for the public version. They help these organizations to work efficiently on numerous projects.
Private 5G also allows organizations to connect various devices with latency as low as 1 millisecond. This helps them access any kind of data in seconds. Moreover, it also gives businesses the opportunity to power IoT and big data projects.
5G also provides financial enterprises to use different commercial 5G carrier services like the low band, mid-band, and high band.
#
Business Benefits of Enterprise 5G Network ArchitectureWith its low latency and great speed, 5G can provide great benefits to financial enterprises. Here is a list of financial enterprises that will benefit from private 5G.
#
Health CareNo other enterprise will benefit from 5G more than hospitals. After Covid broke, a rapid increase in demand for infrastructure emerged. After this medical emergency for the first time in decades, it was noticed that hospitals are short on ICU beds and other technologies required to treat patients. This shortage of beds and basic tech led to the death of thousands of people.
But with private 5G and its seamless connectivity and fast M2M data sharing, future breakouts can be prevented and many diseases can be cured on time. This technology will also minimize the number of deaths.
#
ManufacturingMost manufacturing plants are dependent completely on tech, some wired and some wireless. With 5G, this financial service sector can be fully automated with robots operating manufacturing plants and warehouses. This innovation is not possible with regular wifi or a 4G network because they are limited. While private 5G provides fast speed, connectivity, and low latency that will help improve efficiency in these manufacturing plants.
Private 5G opens gates to endless possibilities of advancement in the manufacturing sector like powerful IoT and a cloud computing system. Moreover, it will also help different financial enterprises increase their production.
#
Automated StoresPrivate 5G also provides opportunities for fully automated stores to improve their user experience with a fast M2M connection. With private 5G, data can be processed many times faster than with 4G, and results can be delivered in seconds. Fully automated store networks can greatly benefit from private 5G network architecture. As these stores work on real-time user input and 5G makes the real-time connection between devices 100 times better than 4G.
Moreover, these stores can also integrate AR or VR technologies in their stores to further enhance the user experience.
#
LogisticsLogistics is another area where financial enterprises can benefit from private 5G. All e-commerce businesses use logistics to track the behavioral pattern of their consumers. Moreover, logistics are also used to track down every step of the product from product dispatch to delivery. All of these logistics work can be more efficiently done using 5G.
This technology will also help businesses automate their facilities and get rid of extra workforce. The automated devices with Private 5G will be more efficient.
#
Smart FacilitiesPrivate 5G is also beneficial to use in smart facilities like airports, offices, malls, etc. These facilities can benefit from ultra-low latency, fast speed, and seamless connectivity. Private 5G will not only improve the overall IT infrastructure of these facilities but also enhance the customer experience.
Private 5G will also enable these smart facilities to stream smooth 4K in real-time without any delay.
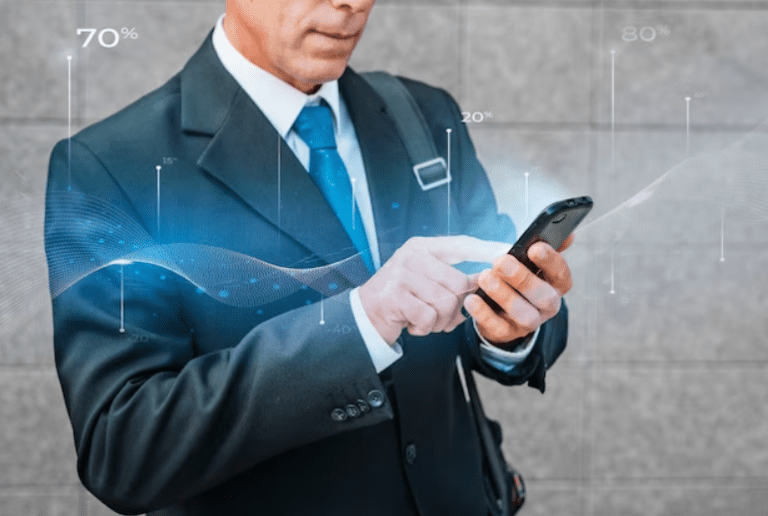
#
ConclusionPrivate 5G is going to innovate the big organization. The infrastructure of big companies will be revolutionized with it. It will provide all the big enterprises with such seamless connectivity, high speed, and ultra-low latency that all of their operations will be completed in the blink of an eye.
Private 5G is different from public 5G; it provides its users with security and many different customization options. It helps different financial enterprises benefit from its connectivity, speed, and latency. It has various useful applications in healthcare, manufacturing, IT, and smart facilities.