How does cloud computing affect budget predictability for CIOs?
Cloud computing companies may assist IT executives in laying the groundwork for success, such as increasing deployment speed and assuring future flexibility. However, the landscape is complicated. While technology is rapidly changing the corporate landscape, technology investment procedures have not always kept up. Let's look at how cloud computing may affect CIO budget predictability.
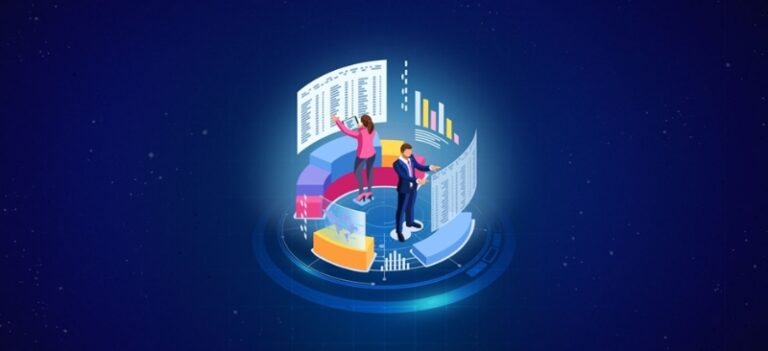
#
Role of CIOs in Cloud Budget PredictabilityCIOs will need to remain up to date on the newest innovations to make the best decisions on behalf of their businesses to drive their digital transformation. Because of the cloud's influence, as well as the DevOps movement, software development and IT operations have been merged and simplified. As infrastructure and applications are no longer independent, the CIO is no longer required to manage manual IT chores [(Makhlouf, 2020)]. Cost-effectiveness and efficiency must be prioritised in their strategy to save cloud budgets, which will bring a new dimension to their conventional job inside a company.
CIOs must also become more adaptable and agile. There are now so many distinct cloud providers that enterprises must employ a multi-access edge computing-cloud approach.
This implies:
- Businesses will be free to select cloud solutions based on their merits rather than being dependent on a single source.
- The CIO will be in charge of expanding a multi-access edge computing-cloud strategy, which means they must think about things like security, service integration, and cost.
Cloud computing companies will increasingly rely on their CIO to develop useful solutions to support digital transformation as cloud computing platforms evolve. As demand grows more than ever, businesses will have a broader selection of cloud-based solutions to choose from. As a result, the CIO's function will be expanded to include both technical expertise and business-oriented strategic thinking.
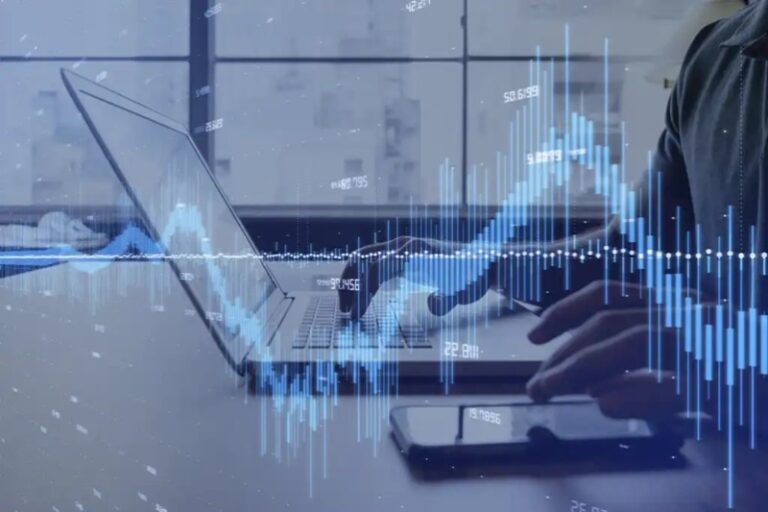
#
CIOs Perspective: From Cost to InvestmentCIOs have long struggled with the impression of IT as a cost centre. The convergence of technology and business strategy might provide CIOs with the chance to abandon a cost-cutting attitude in favour of an investment philosophy that values strategic expenditure to boost revenue, growth, stock price, or other measures of company and shareholder value.
As the technology function assumes a more prominent role, CIOs may need to address critical issues such as core modernization, cloud business models, investment governance and value measurement, the incompatibility of fixed budgets with Agile development, and the impact of automation on the workforce to save cloud budgets [(Liu et al., 2020)].
#
Cloud Computing Affecting Core ModernizationMany CIOs acknowledge that old core systems lack the agility required to build and scale creative and disruptive new technology solutions. Legacy systems can be rehosted, re-platformed, rearchitected, rebuilt, or replaced—strategies that vary in impact, cost, risk, and value. However, core modernization should be considered as a technological investment with other options. A big distribution company's CIO opted to postpone a modernization initiative and shift funding to a bespoke warehouse management program that provided the firm with a competitive edge.
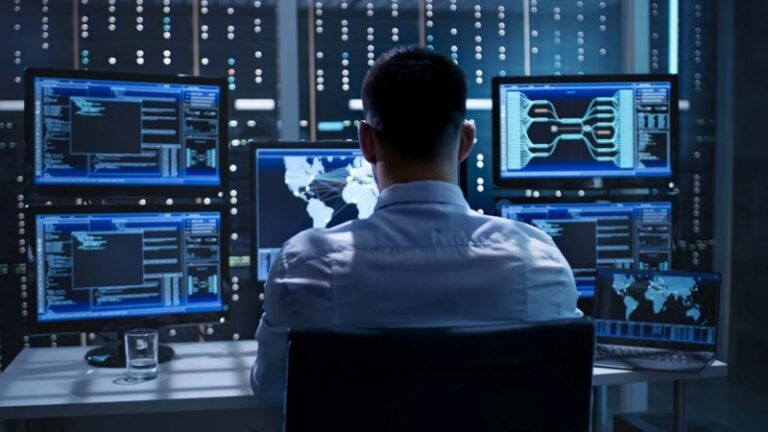
#
Cloud Business Models on OPEX/CAPEXCloud computing companies have welcomed cloud solutions with open arms, drawn by their ease of use and deployment. Cloud computing platforms may foster innovation and encourage experimentation by removing the burden of purchasing and maintaining technological infrastructure [(Kholidy, 2020)]. However, every investment involves risks, and cloud computing platforms are no exception. Because the cloud switches technology spending from the capital expense column to the operating expense column, rushing to the cloud might have a significant impact on firm financials. Finance and IT divisions may collaborate to properly identify these expenses and analyze and maximize the impact to save cloud budgets.
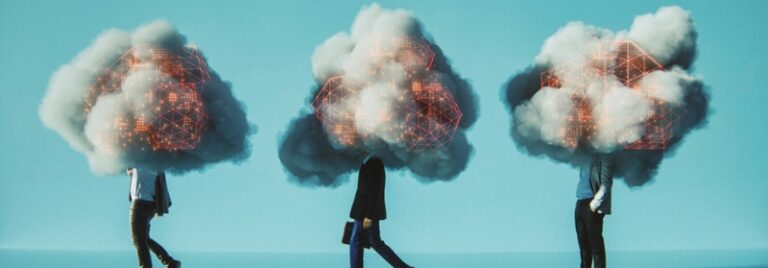
#
Governance and Value AssessmentTechnology leaders may improve their capacity to create convincing business cases that properly anticipate technology project ROI and assess the performance and value of each investment [(Liu et al., 2018)]. It can be beneficial to have a specialized financial team responsible for modeling, administering, and analyzing the value of IT investments to save cloud budgets.
Taking such actions can help decrease the notion that technology is an incomprehensible black box, make it simpler for technology executives to justify spending, and help them create closer connections with CFOs.
#
Incompatibility of Fixed BudgetsAgile and other flexible delivery techniques are on the increase. CIOs may manage investment portfolios in the same way that venture capitalists do, but only if financing mechanisms are changed to favor Agile, product-focused settings. A flexible budgeting methodology may provide product teams with the necessary creativity and responsibility to achieve business value and save cloud budgets.
#
Automation ImpactAutomation and robotics' ability to streamline and accelerate IT delivery is changing the way technology and cloud computing companies work, collaborate, and create value [(Raj and Raman, 2018)]. Better workflows and various resource needs might drive increased production output and save cloud budgets as automation enables teams to exchange manual and repetitive jobs for those requiring higher-order abilities.